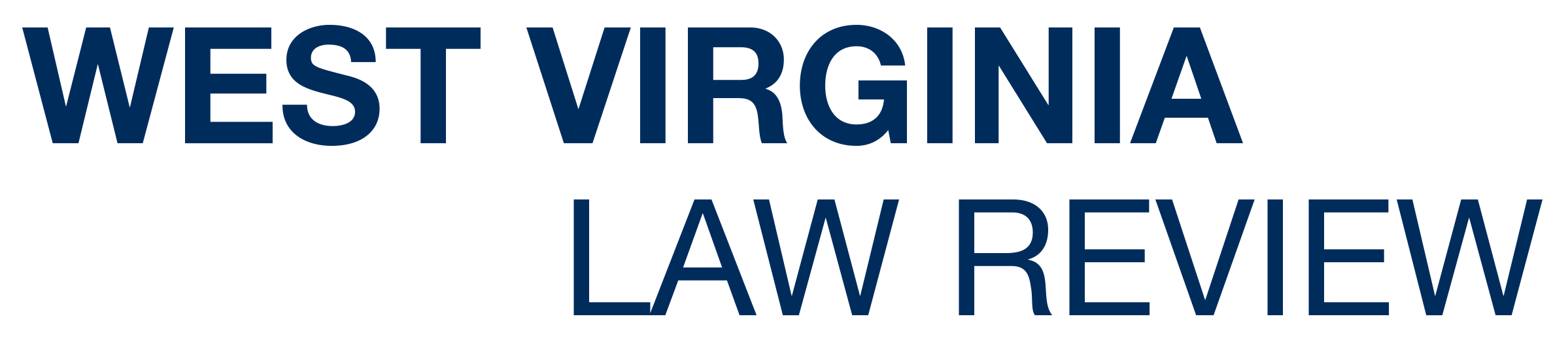
Document Type
Article
Abstract
Artificial intelligence is based, in part, on learning algorithms that can continually monitor and embed new data from large numbers of sources to create ever "improved" decisions, whether the decisions are applied to the physical world like the operation of smart cars, or whether the decision is about the extension of credit to a loan applicant. Yet, it is well known that algorithms and resulting decision making AI models are plagued by unintended bias and discriminatory results. Data science scholars have attempted to address bias and discrimination through the imposition of multiple mathematically represented options to measure fairness. However, these mathematical measures can conflict, and they can be incompatible with legal concepts of fairness, especially those found in non-discrimination laws. This article provides an introduction to the multiple meanings of fairness in both the data science and legal disciplines and uses a case study of AI and alternative data credit scoring to illustrate how the use of different disciplinary meanings of fairness will significantly affect societal outcomes. In conclusion, it is proposed that a socio-technical approach to AI fairness, which incorporates legal concepts, will increase the acceptance, legitimacy, and trust of those systems.
Recommended Citation
Janine S. Hiller,
Fairness in the Eyes of the Beholder: AI; Fairness; and Alternative Credit Scoring,
123
W. Va. L. Rev.
907
(2021).
Available at:
https://researchrepository.wvu.edu/wvlr/vol123/iss3/8